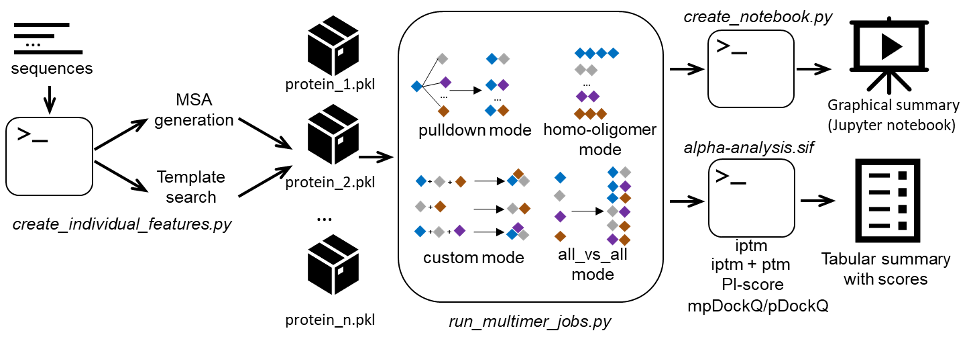
AlphaPulldown and PBS Job Array Demo
Ku Wee Kiat, Research Computing, NUS IT
AlphaFold2 has enabled structural modelling of proteins with accuracy comparable to experimental structures. A variety of modifications of AlphaFold2 have been developed to facilitate specific applications such as ColabFold (Mirdita et al., 2022), which accelerates the program and exposes useful parameters. AlphaFold-Multimer can also be applied to screen large datasets of proteins for new protein-protein interactions (PPIs) (Humphreys et al., 2021; Bryant, Pozzati, and Elofsson, 2022) and to model combinations and their fragments when modelling complexes (Mosalaganti et al., 2022).
Alphapulldown was developed as a Python package that streamlines PPIs screens and high-throughput modelling of higher-order oligomers using AlphaFold-Multimer.
- Provides a convenient command line interface to screen a bait protein against many candidates, calculate all-versus-all pairwise comparisons, test alternative homo-oligomeric states, and model various parts of a larger complex
- Separates the CPU stages (MSA and template feature generation) from GPU stages (the actual modeling)
- Allows modeling fragments of proteins without recalculation of MSAs and keeping the original full-length residue numbering in the models
- Summarises the results in a CSV table with AlphaFold scores, pDockQ and mpDockQ, PI-score, and various physical parameters of the interface
- Provides a Jupyter notebook for an interactive analysis of PAE plots and models
Demonstration
Example 1 from the AlphaPulldown git repository will be demonstrated in this article. The aim of example 1 is to find proteins involving human translation pathway that might interact with eIF4G2.
Example 1 contains 3 steps:
- Compute multiple sequence alignment (MSA) and template features (run on CPUs)
- Predict structures (run on GPU)
- Evaluation and visualisation
Step 1: Compute multiple sequence alignment (MSA) and template features (run on CPUs)
Source: Link
Below is the job script for running step 1 of the example. Note that it launches an array of PBS jobs using the directive “#PBS -J 1-21:1”.
What it does is to split the pairs of the bait and 21 candidate sequences into individual jobs and queue them in one go. The AlphaPulldown is 1-indexed so the range of numbers starts with 1 to 21. The number 21 is derived from the following calculation:
baits=`grep ">" baits.fasta | wc -l`
candidates=`grep ">" example_1_sequences_shorter.fasta | wc -l`
count=$(( $baits + $candidates ))
PBS Job Array
The inclusion of the PBS directive #PBS -J n-m:step (where n is the starting index, m is the ending index, and the optional step is the step size) will state to PBS that this PBS script is a job array, and PBS will queue this script in “FLOOR[(m-n)/step+1]” instances. The environment variable $PBS_ARRAY_INDEX will contain the current index instance your script is in.
When you submit a job array PBS job, the PBS job number will have left and right brackets, “[]”, appended to it. A job that would normally look something like “5141439”, would now look like “5141439[]” in the PBS qstat command.
Job Script
#!/bin/bash
#PBS -P alphafold_project_name
#PBS -j oe
#PBS -N alphapulldown_array_shorter
#PBS -q parallel20
#PBS -l select=1:ncpus=20:mem=100gb:cluster=atlas9
#PBS -l walltime=720:00:00
#PBS -J 1-21:1
## have to precompute the total number
# Precompute this and indicate above for PBS -J
#baits=`grep ">" baits.fasta | wc -l`
#candidates=`grep ">" example_1_sequences_shorter.fasta | wc -l`
#count=$(( $baits + $candidates ))
JOBID=`echo ${PBS_JOBID} | cut -d'[' -f1`
JOBDATE=$(date +%Y-%m-%d --date="today")
cd $PBS_O_WORKDIR;
np=$(cat ${PBS_NODEFILE} | wc -l);
source /etc/profile.d/rec_modules.sh
module load singularity
IMAGE="/app1/common/singularity-img/3.0.0/user_img/alphapulldown_v0.21.6.sif"
###
ALPHAFOLD_DATA_PATH=/scratch2/biodata/alphafold/database/
OUTPUT_DIR=`pwd`/output/${JOBID}_${JOBDATE}_apd_out/
mkdir -p $OUTPUT_DIR
echo $OUTPUT_DIR
singularity exec -e --bind /scratch2 $IMAGE bash << EOF > `echo $OUTPUT_DIR`/stdout.${PBS_ARRAY_INDEX}.$JOBID 2> `echo $OUTPUT_DIR`/stderr.${PBS_ARRAY_INDEX}.$JOBID
create_individual_features.py \
--fasta_paths='baits.fasta,example_1_sequences_shorter.fasta' \
--data_dir=${ALPHAFOLD_DATA_PATH} \
--mgnify_database_path=$ALPHAFOLD_DATA_PATH/mgnify/mgy_clusters.fa \
--output_dir=${OUTPUT_DIR} \
--save_msa_files=False \
--use_precomputed_msas=False \
--max_template_date="2022-03-30" \
--skip_existing=True \
--seq_index=${PBS_ARRAY_INDEX}
EOF
To look at the indices that are remaining:
$ qstat -f 5141439[] | grep array_indices |
For the current status of the job array:
$ qstat -f 5141439[] | grep array_state |
For the status of jobs:
$ qstat -swu ccekwk
venus01:
Req'd Req'd Elap
Job ID Username Queue Jobname SessID NDS TSK Memory Time S Time
------------------------------ --------------- --------------- --------------- -------- ---- ----- ------ ----- - -----
5141439[].venus01 ccekwk parallel20 alphapulldown_* -- 1 20 100gb 720:0 B --
Job Array Began at Mon Nov 28 at 16:18
Output when job completes:
$ ls output/5141439_2022-11-28_apd_out/
O76094_feature_metadata_2022-11-28.txt P62945_feature_metadata_2022-11-29.txt stderr.12.5141439 stdout.13.5141439
O76094.pkl P62945.pkl stderr.13.5141439 stdout.14.5141439
P08240_feature_metadata_2022-11-28.txt P78344_feature_metadata_2022-11-28.txt stderr.14.5141439 stdout.1.5141439
P08240.pkl P78344.pkl stderr.1.5141439 stdout.15.5141439
P09132_feature_metadata_2022-11-28.txt Q14240_feature_metadata_2022-11-28.txt stderr.15.5141439 stdout.16.5141439
P09132.pkl Q14240.pkl stderr.16.5141439 stdout.17.5141439
P22090_feature_metadata_2022-11-28.txt Q7L2H7_feature_metadata_2022-11-28.txt stderr.17.5141439 stdout.18.5141439
P22090.pkl Q7L2H7.pkl stderr.18.5141439 stdout.19.5141439
P23588_feature_metadata_2022-11-28.txt Q92901_feature_metadata_2022-11-29.txt stderr.19.5141439 stdout.20.5141439
P23588.pkl Q92901.pkl stderr.20.5141439 stdout.21.5141439
P37108_feature_metadata_2022-11-28.txt Q9BW92_feature_metadata_2022-11-28.txt stderr.21.5141439 stdout.2.5141439
P37108.pkl Q9BW92.pkl stderr.2.5141439 stdout.3.5141439
P46778_feature_metadata_2022-11-29.txt Q9H2W6_feature_metadata_2022-11-28.txt stderr.3.5141439 stdout.4.5141439
P46778.pkl Q9H2W6.pkl stderr.4.5141439 stdout.5.5141439
P46781_feature_metadata_2022-11-29.txt Q9NX20_feature_metadata_2022-11-29.txt stderr.5.5141439 stdout.6.5141439
P46781.pkl Q9NX20.pkl stderr.6.5141439 stdout.7.5141439
P60842_feature_metadata_2022-11-28.txt Q9UHB9_feature_metadata_2022-11-28.txt stderr.7.5141439 stdout.8.5141439
P60842.pkl Q9UHB9.pkl stderr.8.5141439 stdout.9.5141439
P61011_feature_metadata_2022-11-28.txt Q9Y5M8_feature_metadata_2022-11-28.txt stderr.9.5141439
P61011.pkl Q9Y5M8.pkl stdout.10.5141439
P61247_feature_metadata_2022-11-29.txt stderr.10.5141439 stdout.11.5141439
P61247.pkl stderr.11.5141439 stdout.12.5141439
Each candidate sequence will have its own stderr and stdout log files, as well as feature metadata and features (in .pkl).
Step 2: Predict Structures (Run on GPU)
Source: Link
Similar to step 1, we have to derive the parameters for the PBS Job Array with the following,
baits=`grep -c "" baits.txt`
candidates=`grep -c "" candidates_shorter.txt`
count=$(( $baits * $candidates ))
For this example, the PBS Job Array directive would be: #PBS -J 1-20:4, with step size 4, this would create 5 jobs, sequentially running 4 candidates in each job. We would have to request ample wall time for the jobs to complete.
#!/bin/bash
#PBS -P alphafold_project_name
#PBS -j oe
#PBS -N alphapulldown_array_shorter
#PBS -q volta_gpu
#PBS -l select=1:ncpus=10:mem=80gb
#PBS -l walltime=72:00:00
#PBS -J 1-20:4
## have to precompute the total number
# Precompute this and indicate above for PBS -J
#baits=`grep ">" baits.fasta | wc -l`
#candidates=`grep ">" candidates_shorter.fasta | wc -l`
#count=$(( $baits + $candidates ))
JOBID=`echo ${PBS_JOBID} | cut -d'[' -f1`
JOBDATE=$(date +%Y-%m-%d --date="today")
cd $PBS_O_WORKDIR;
np=$(cat ${PBS_NODEFILE} | wc -l);
source /etc/profile.d/rec_modules.sh
module load singularity
IMAGE="/app1/common/singularity-img/3.0.0/user_img/alphapulldown_v0.21.6.sif"
###
ALPHAFOLD_DATA_PATH=/scratch2/biodata/alphafold/database/
INPUT_MONOMER_DIR=`pwd`/output/5141439_2022-11-28_apd_out/
OUTPUT_DIR=${INPUT_MONOMER_DIR}_step2_${JOBID}
mkdir -p $OUTPUT_DIR
echo $OUTPUT_DIR
stepsize=20
current_idx=$PBS_ARRAY_INDEX
max_idx=$(( $PBS_ARRAY_INDEX + $stepsize ))
baits=`grep -c "" baits.txt`
candidates=`grep -c "" candidates_shorter.txt`
count=$(( $baits * $candidates ))
if (( max_idx > count )); then
max_idx=$count
fi
for (( c=$PBS_ARRAY_INDEX; c<$max_idx; c++ ))
do
singularity exec -e --bind /scratch2 $IMAGE bash << EOF > `echo $OUTPUT_DIR`/stdout.${c}.$JOBID 2> `echo $OUTPUT_DIR`/stderr.${c}.$JOBID
echo "Starting number $c Analysis"
export XLA_PYTHON_CLIENT_MEM_FRACTION=2.0
export TF_FORCE_UNIFIED_MEMORY='1'
run_multimer_jobs.py --mode=pulldown \
--num_cycle=3 \
--num_predictions_per_model=1 \
--output_path=${OUTPUT_DIR} \
--data_dir=${ALPHAFOLD_DATA_PATH} \
--protein_lists=baits.txt,candidates_shorter.txt \
--monomer_objects_dir=${INPUT_MONOMER_DIR} \
--job_index=${c}
EOF
done
The INPUT_MONOMER_DIR must be the output directory of the Step 1 job.
When the job completes, these are the outputs that you will see:

Step 3: Evaluation and Visualisation
Source: Link
When a batch of jobs is finished, AlphaPulldown can create a Jupyter notebook that presents an overview of the models.
To generate the notebook, logon to Atlas9 login node, then run the following commands.
module load singularity
IMAGE="/app1/common/singularity-img/3.0.0/user_img/alphapulldown_v0.21.6.sif"
singularity exec -e --bind /scratch2 $IMAGE bash
# Change directory to Step 2’s output directory
cd /scratch2/ccekwk/alphapulldown/output/5141439_2022-11-28_apd_out/_step2_5142236
create_notebook.py --cutoff=5.0 --output_dir=.
A file named output.ipynb will be created.
To view the file, in the same terminal window and same folder, run
jupyter-lab output.ipynb
You should be able to see a link that starts with http://localhost:8888 etc. You will need this link later.
Next, open another terminal window and run the following command to forward the Jupyter Lab instance to your local computer.
ssh -L 8888:localhost:8888 nusnet_id@atlas9
Open a browser and go to http://localhost:8888/lab?token=[see first terminal for the token]
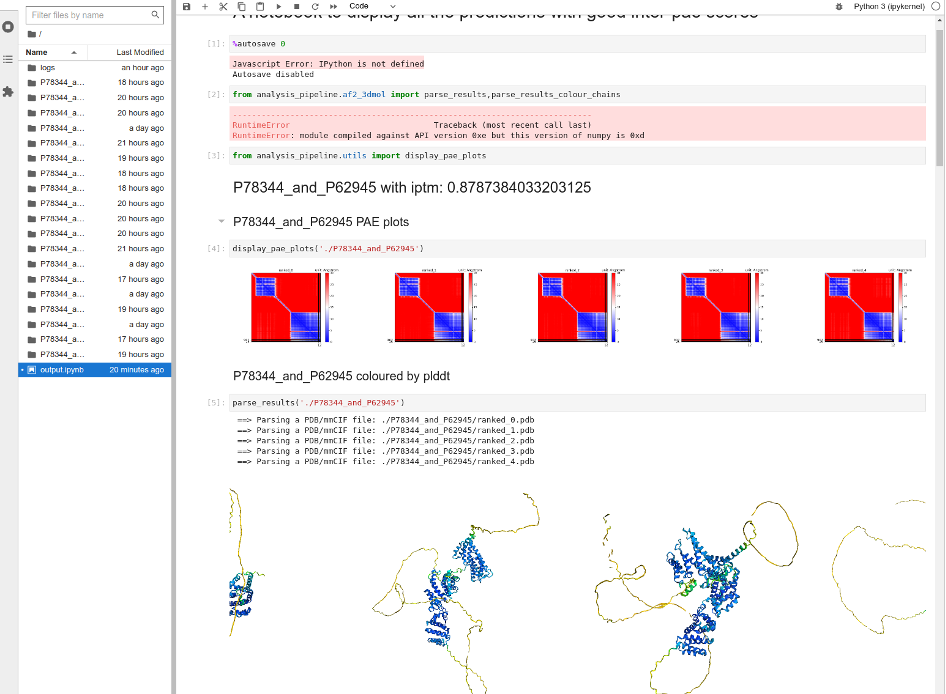
If you encounter any issues, please raise a HPC Enquiry on www.ntouch.nus.edu.sg